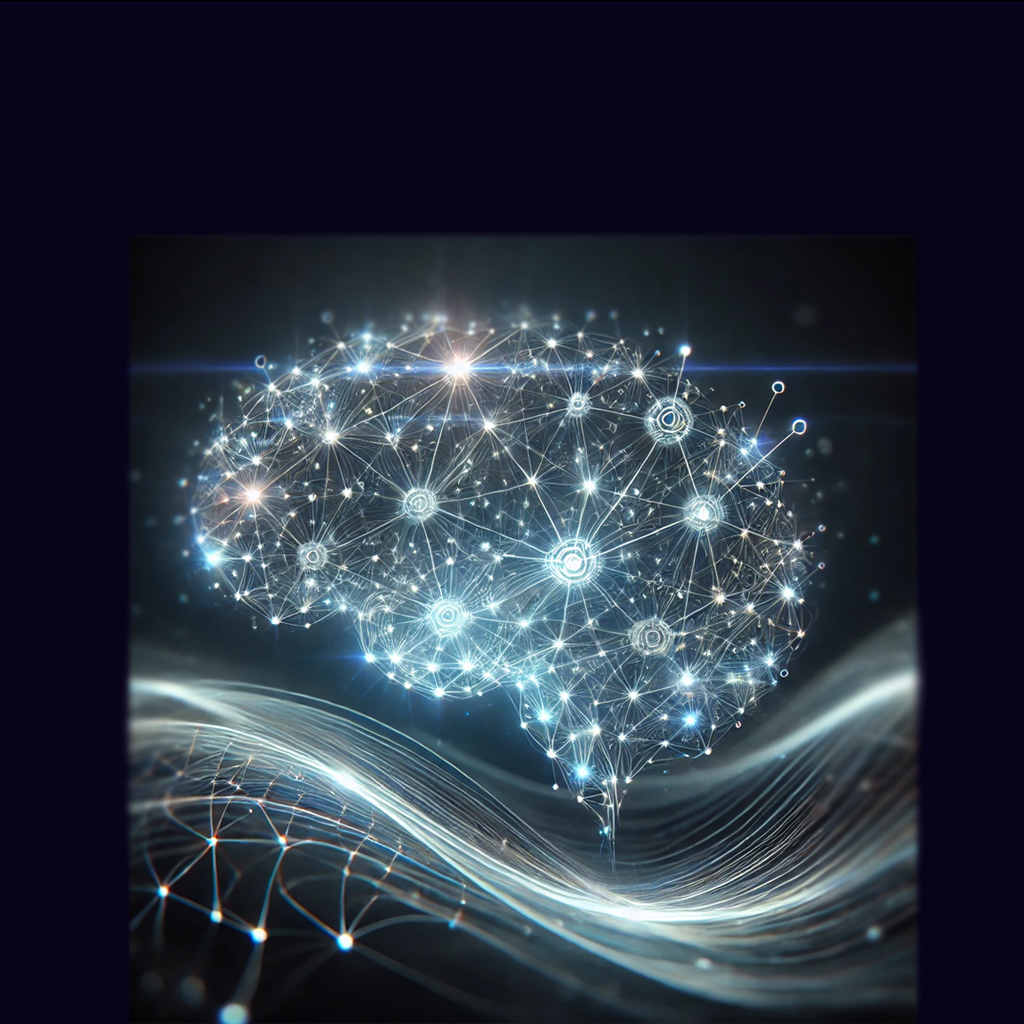
This week, on 8 October 2024, it was announced that the 2024 Nobel Prize in Physics has been awarded to John J. Hopfield and Geoffrey E. Hinton “for foundational discoveries and inventions that enable machine learning with artificial neural networks”.
Their groundbreaking work laid the foundations for modern machine learning through the development of artificial neural networks. Their contributions have profoundly influenced various fields, from artificial intelligence to materials science, showcasing the intersection of physics and computer science.
John J. Hopfield is recognized for creating the Hopfield network, a type of recurrent artificial neural network that functions as an associative memory system capable of storing and reconstructing patterns within data. The network can store patterns by adjusting the weights between neurons and later retrieve these patterns even when presented with partial or noisy inputs. This ability makes Hopfield networks useful for image processing, pattern recognition, optimization problems, and error correction enabling machines to interpret incomplete data accurately.
Geoffrey Hinton, often referred to as one of the “godfathers of deep learning” built upon Hopfield’s work by developing the Boltzmann machine, which is a type of stochastic recurrent neural network. It consists of symmetrically connected neurons that operate in a binary state. Unlike deterministic models, Boltzmann machines incorporate randomness in their operation, making decisions based on a probability distribution. They are capable of learning internal representations and solving combinatorial optimization problems. Although computationally intensive in their original form, Boltzmann machines have been influential in the development of deep learning architectures.
The Hopfield network and Boltzmann machine are pivotal models in the evolution of artificial neural networks and machine learning. These models provided crucial insights into associative memory, collective computation, and probabilistic modelling. The Hopfield network demonstrated how simple interconnected units could store and retrieve patterns, mimicking aspects of human memory. The Boltzmann machine introduced stochastic elements, bridging neural networks with concepts from statistical physics. Both models utilized energy-based frameworks, which proved influential in understanding network dynamics and optimization.
The significance of Hopfield and Hinton’s achievements lies not only in their theoretical contributions but also in the practical implications of their discoveries. Today, artificial neural networks underpin much of the technology we use daily, from virtual assistants to self-driving cars. Their work exemplifies how principles from physics can be applied to solve complex problems in other domains, fostering interdisciplinary collaboration.
At GJE, our Computer and Software based team has extensive experience in patenting machine learning and AI-based inventions and navigating the increasingly complex intellectual property landscape. To discuss your AI/ML strategy, please get in contact with us at gje@gje.com.